As our population grows and ages, so do cancer deaths. The good news is that age-adjusted cancer deaths have actually fallen since 1990, though only by a measly 15.22% as of 2019 (Figure 1). This is despite literally hundreds of new cancer therapeutics hitting the market in that time. So why the disappointing improvement to survival? As the World Health Organization puts it, it is early diagnosis that saves lives and cuts treatment costs. The key to screening is non-invasive, reliable, scalable tests that can detect cancer at an early stage of pathogenesis, allowing for swift treatment. Whilst that sounds like a wishlist of potentially unrealistic proportions, recent research suggests that there could be an answer: extracellular vesicles (EVs). These tiny parcels of proteins, nucleic acids and small molecules are released by all cells – including cancer cells – into bodily fluids.
The interest in EVs as cancer diagnostics has grown exponentially in recent years, with >150 times as many articles published on the topic in 2022 than in 2011 (source: Web of Science). Given the potential for EVs to shuttle cancer-derived proteins, RNAs or even DNA to biofluids which are accessible for non-invasive diagnosis, the potential for diagnostics in this space is immense. However, many of the methods used in the development of these diagnostic tests are suboptimal as they do not isolate EVs with sufficient purity and may not be scalable to a diagnostic setting – let alone a large screening programme. In this article, we take a look at three publications from within the EV cancer diagnostics space, each comparing different EV isolation methods.
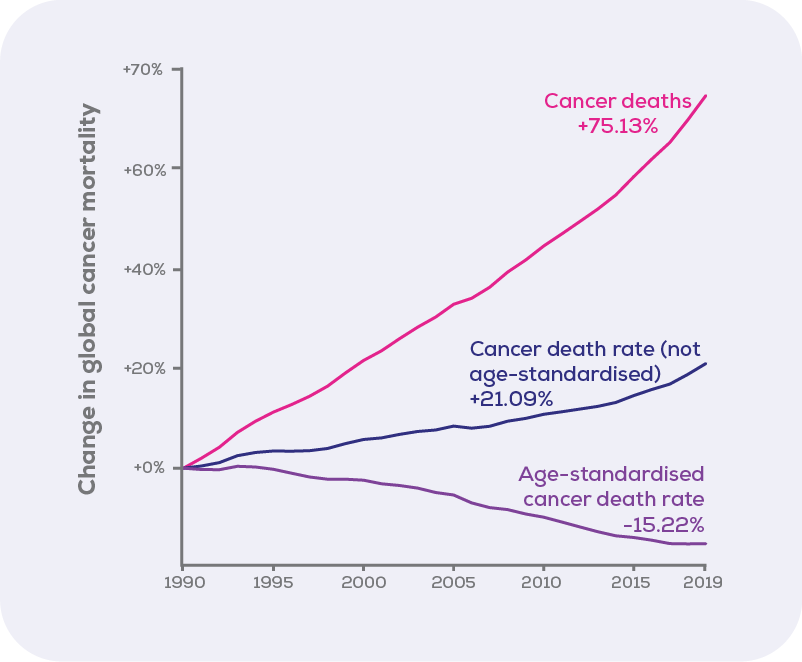
3 studies comparing EV isolation methods for cancer diagnostics
The first of these three studies is by Pang et al. (2020)1, which hails from the field of prostate cancer diagnosis where the current gold diagnostic standard of prostate specific antigen leads to many unnecessary biopsies.2 In the study by Pang et al., they aimed to determine whether plasma EVs of prostate origin could harbour more specific biomarkers.
The second study is by Northrop-Albrecht et al. (2022)3 who turned to human stool supernatant EVs for the diagnosis of colon cancer. Early diagnosis of colon cancer is particularly important, as late diagnosis turns a 91% 5 year survival rate for localised cancer into just a 13% survival rate for cancer with distal spread.
Finally, the study by Anastasi et al. (2020)4 looks at a mouse model of glioblastoma multiform, with the hope of perfecting serum EV isolation techniques to allow for the identification of longitudinal markers of disease progression.
Principles behind the EV isolation methods compared
The methods compared in each study are shown in Figure 2.
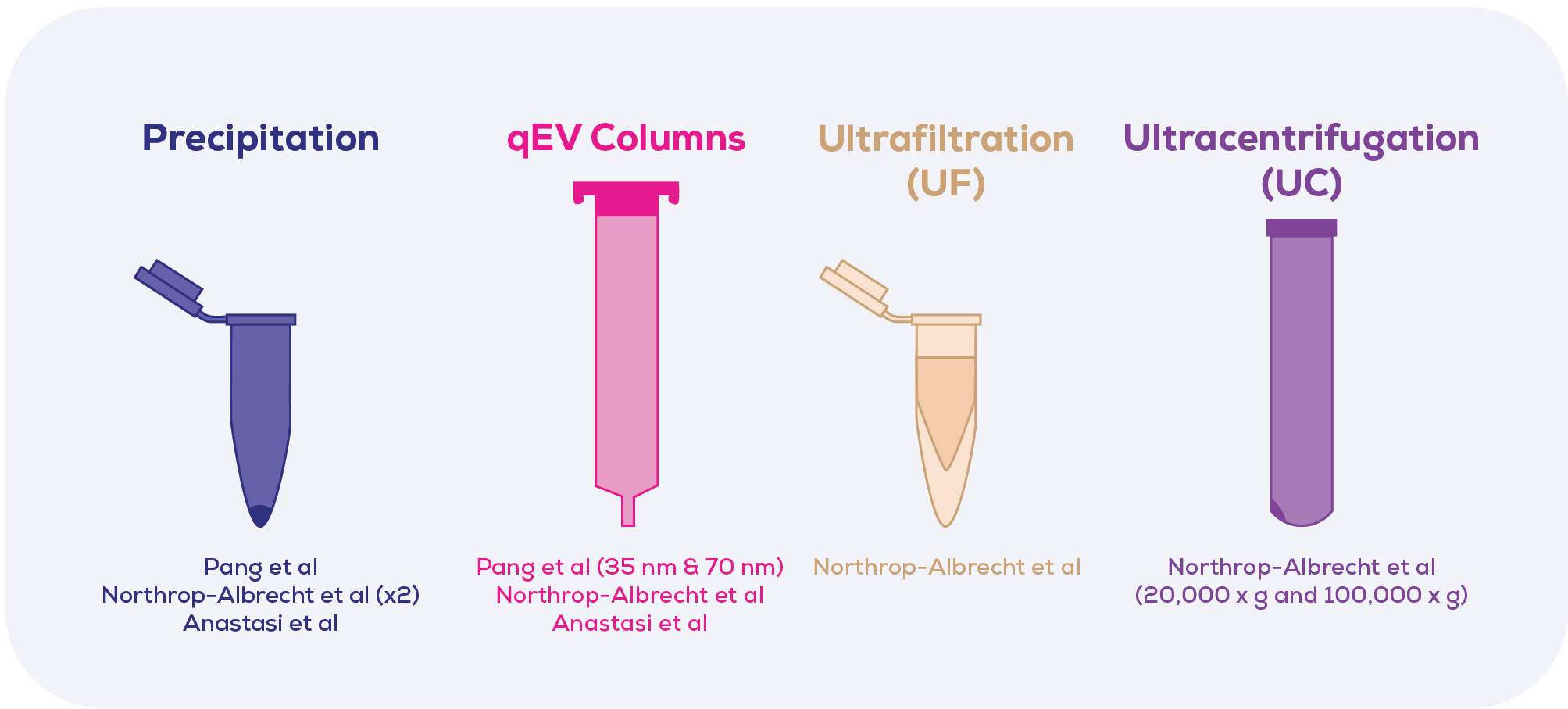
Precipitation utilises polymerisation of a polymer such as polyethylene glycol (often abbreviated to PEG) to form a mesh in which larger particles – and, unfortunately lots of smaller ones – are trapped. This mesh can then be centrifuged down to the bottom of the tube. Whilst nothing about this procedure is specific for EVs, it is frequently sold and used as if it is.
Our own qEV columns use size exclusion chromatography to separate EVs from other particles based upon their size. qEV columns come in a variety of sizes, allowing for samples from 150 µL to 100 mL to be isolated based upon the same principles. qEV columns come in two ranges: the 35 nm and 70 nm Series, which isolateslightly different EV size ranges.
Ultrafiltration concentrates EVs and any other larger molecules from the starting sample into a smaller volume and, like precipitation, does not truly isolate EVs on its own.
Ultracentrifugation is the gold standard in the EV field. It uses increasing centrifugal force to pellet different sized particles, with EVs generally being pelleted at or above 100,000 x g. Whilst regarded as the gold standard, this technique is increasingly falling out of favour due to a lack of purity, long processing times and the force-driven damage of EVs.
So, how did these methods compare in the context of cancer diagnostics?
Of these methods, qEV columns isolate the purest EVs
Contamination with non-EV components can swamp the signals of true cancer EV biomarkers, preventing biomarker discovery, validation, and crucial early diagnosis. For EV-associated protein biomarkers, this means that soluble proteins and lipoproteins must be depleted. Whilst for an EV-RNA biomarker, non-EV RNA depletion is key.
qEV columns excel at removing protein contamination
The degree of contamination with soluble proteins in EV isolates is often displayed by the ratio of EVs to protein concentration. Both Pang et al. and Anastasi et al. found that qEV columns resulted in isolates with a higher EV:protein ratio than precipitation from plasma and serum (Figure 3A and C). qEV 70 nm columns slightly outperformed 35 nm columns, which makes sense as 35 nm columns have a smaller size cut-off, meaning that some larger protein complexes may be co-isolated. Northrop-Albrecht et al found that ultracentrifugation may offer a slightly higher purity than other techniques for stool supernatant samples, though the variation in purity for ultracentrifugation was very high (Figure 3B).
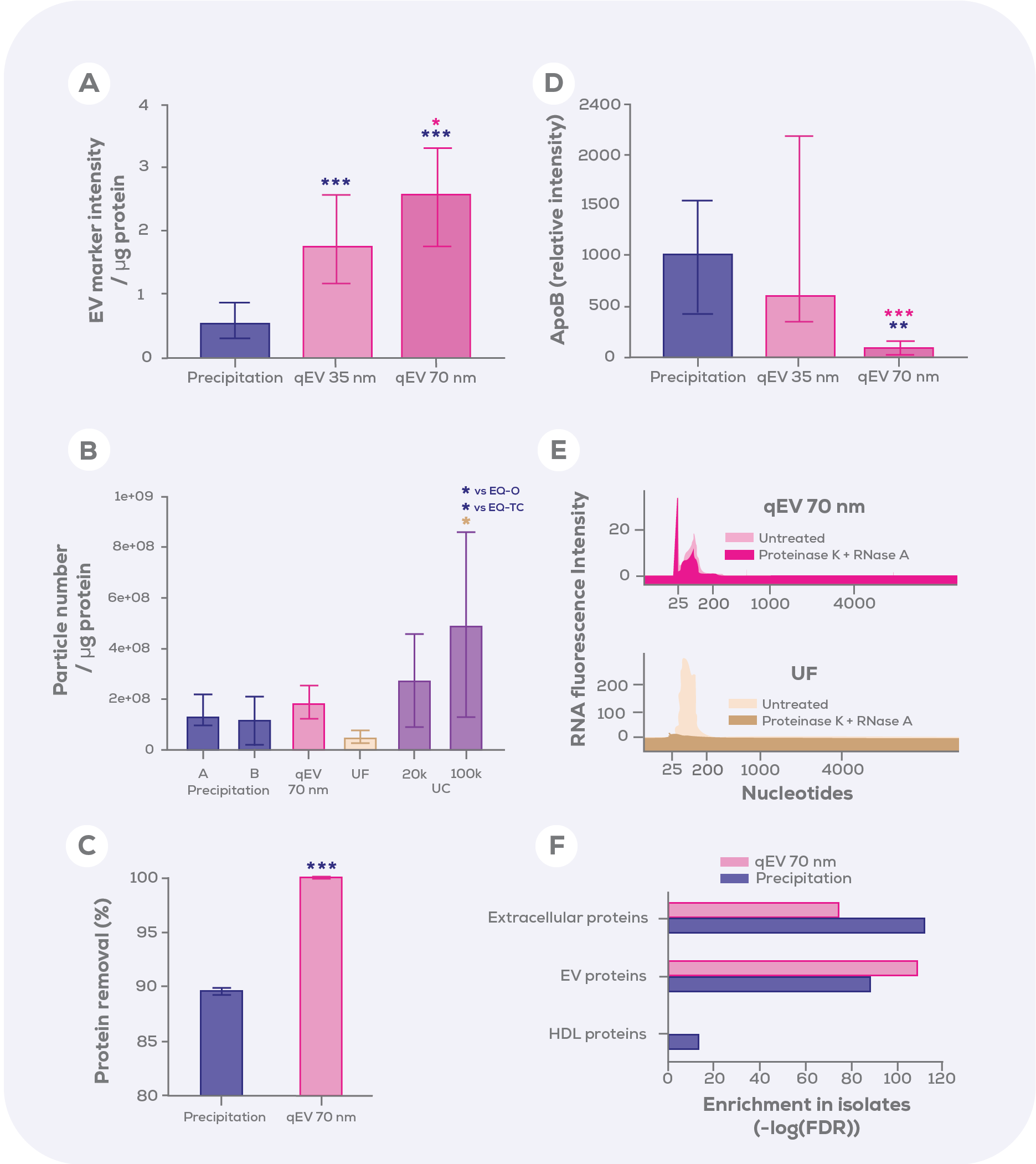
A and D: Pang et al., determined the isolate purity with regards to protein contamination (A; relative intensity on bead array of EV markers CD81, CD9 and CD63 divided by µg of protein in isolates) and lipoprotein contamination (D; ApoB intensity in isolates) in EVs from the plasma of prostate cancer patients or healthy controls isolated using a commercial precipitation kit, or qEV 35 nm or 75 nm columns. Statistical analysis was by One-way ANOVA with an unspecified post-hoc test.
B and E: Northrop-Albrecht et al., determined the purity in terms of protein contamination (B; particle number per µg of protein) and RNA contamination (E; degradation of non-EV RNA in isolates following proteinase K and RNase A treatment) for EVs isolated using a variety of methods from human stool for colorectal cancer diagnostics. UF = ultrafiltration; UC = ultracentrifugation; 20k = 20,000 x g pellet; 100k = 100,000 x g pellet. Precipitation A and B represent two different commercial precipitation kits. Statistical analysis was by a Kruskal-Wallis test.
C and F: Anastasi et al., determined the purity in terms of percentage protein removal in isolates (C; calculated from protein concentration in isolates divided by the 5,000µg/µL in starting material) and relative enrichment of contaminant or ‘exosome’ proteins (F; DAVID enrichment analysis) of EVs isolated from a murine model of glioblastoma multiforme using a commercial precipitation kit and qEV 70 nm. Statistical analysis was by paired T-test (C) or Fisher's exact test with false discovery rate (FDR) multiple testing correction.
* p<0.05; ** p<0.01; *** p<0.001
Lipoprotein contamination is reduced by qEV columns
As they were using plasma and serum respectively, Pang et al. and Anastasi et al. were also interested in lipoprotein contamination. Pang et al. found that ApoB contamination was far lower with qEV 70 nm columns than for precipitation. qEV 35 nm columns showed a level of ApoB comparable with precipitation, likely due to the lower size cut-off of these columns. Anastasi et al. used proteomics to determine that whilst there was no enrichment of lipoproteins in qEV 70 nm isolations, precipitation isolates were enriched for lipoproteins. These data show that qEV 70 nm columns are superior at removing lipoprotein contamination as compared to the commonly used precipitation method.
qEV columns drastically reduce non-EV RNA contamination
Regarding non-EV RNA contamination, qEV 70 nm columns also performed best, with only a small difference between the RNA profile of qEV 70 nm isolates following treatment with proteinase K and RNase A to degrade non-EV RNA (Figure 3E). The same could not be said for ultrafiltration isolates, which isolated a large amount of non-EV RNA which would obscure the identification or measurement of EV RNA cancer biomarkers.
When it comes to scalability, the qEV column wins hands down
For a diagnostic test to be successful, it must be scalable. This means that ultracentrifugation is immediately discounted due to isolation processing times which often exceed 3 hours per sample. That’s before any biomarker measurement can occur. Ultracentrifugation also requires large, expensive and – if used incorrectly – highly dangerous equipment. Ultrafiltration has the potential for scalability and automation, but the attractiveness of solely using ultrafiltration to isolate EVs for diagnostics is limited by the relative impurity of the technique (Figure 2E).
By far the quickest method requiring the least skill to perform is precipitation, which has led to this technique being used in diagnostic research and trials. However, it is highly non-selective for EVs and shows poor removal of contamination (Figure 2A, C, D and F). Could the use of precipitation in diagnostic studies be hampering the discovery process? Given these results, this is a definite possibility.
On the other hand, qEV columns are highly scalable, and columns are available in a range of sizes to suit all sample types. Additionally, we offer a high degree of automation of the EV isolation process with qEV columns, allowing for standardised isolations ideal for delivering a high-quality diagnostic test.
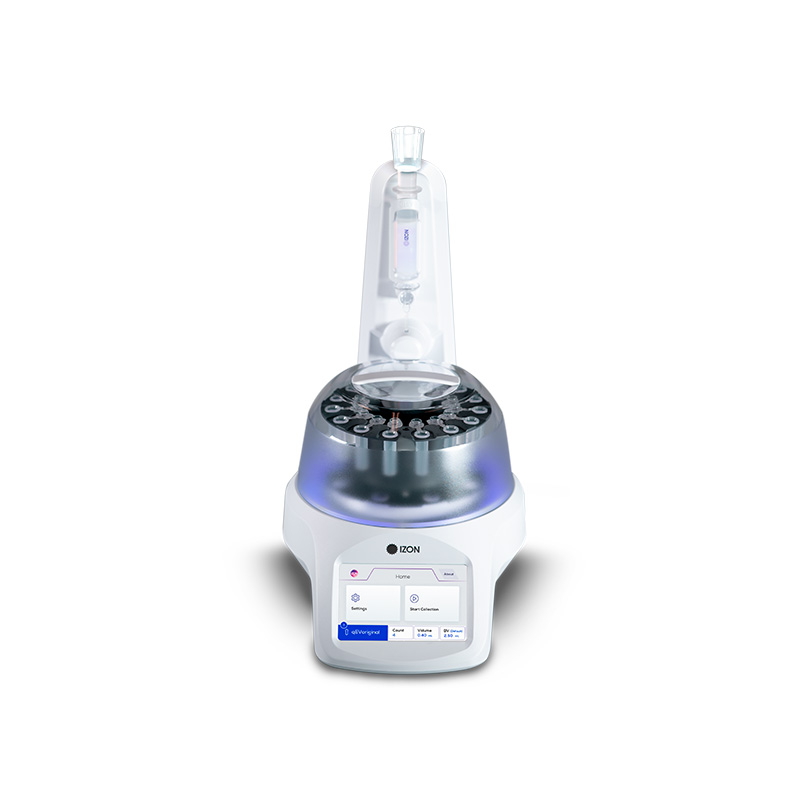
Which isolation method should you pick for cancer diagnostics?
Of course we’re biased, we do make the qEV columns. But the science does back us up – and EV diagnostics researchers are turning to size exclusion chromatography, largely for its high level of scalability and reproducibility over other methods. The use of qEV columns allows for standardised, automated isolation of pure EVs from clinical samples for diagnostic purposes. With better purity and the replicability that comes from automation and good quality products, using the qEV Isolation system will ensure that you are in the best position to identify meaningful biomarkers to ensure that patients get the early diagnosis that they need.
Indeed, our qEV columns have already been used in numerous studies by researchers in the cancer diagnostics field. For example, Mercy Bioanalytics utilise our qEV columns in their Mercy Halo™ diagnostic tests, as described in a recent preprint focused on detecting early-stage ovarian tumours in human plasma.5 In the field of non-small lung cancer, qEV columns have been used by the groups of David P. Carbone6 and Andreas Möller7 to track disease trajectory and response to treatment. In breast cancer, qEV columns have been used by Sima Lev’s group to isolate EVs to analyse the disease progression and response to treatment8, by Francesco Fabbri’s group to isolate EVs for the identification of early diagnostic biomarkers9, and by the groups of Clotilde Théry and Charlotte Proudhon to identify protein biomarkers of metastatic disease10. In the study of prostate cancer, qEV columns feature in work by Rienk Nieuwland’s group at Amsterdam UMC11, as well as by Karla C. Williams and her group12 in the identification of potential plasma biomarker STEAP1. These few examples are only a tiny fraction of the body of literature where qEV columns have been used by cancer researchers.
Keen to learn more? Get in touch to find out how we can help you scale up and refine your diagnostics throughput (including customised solutions).